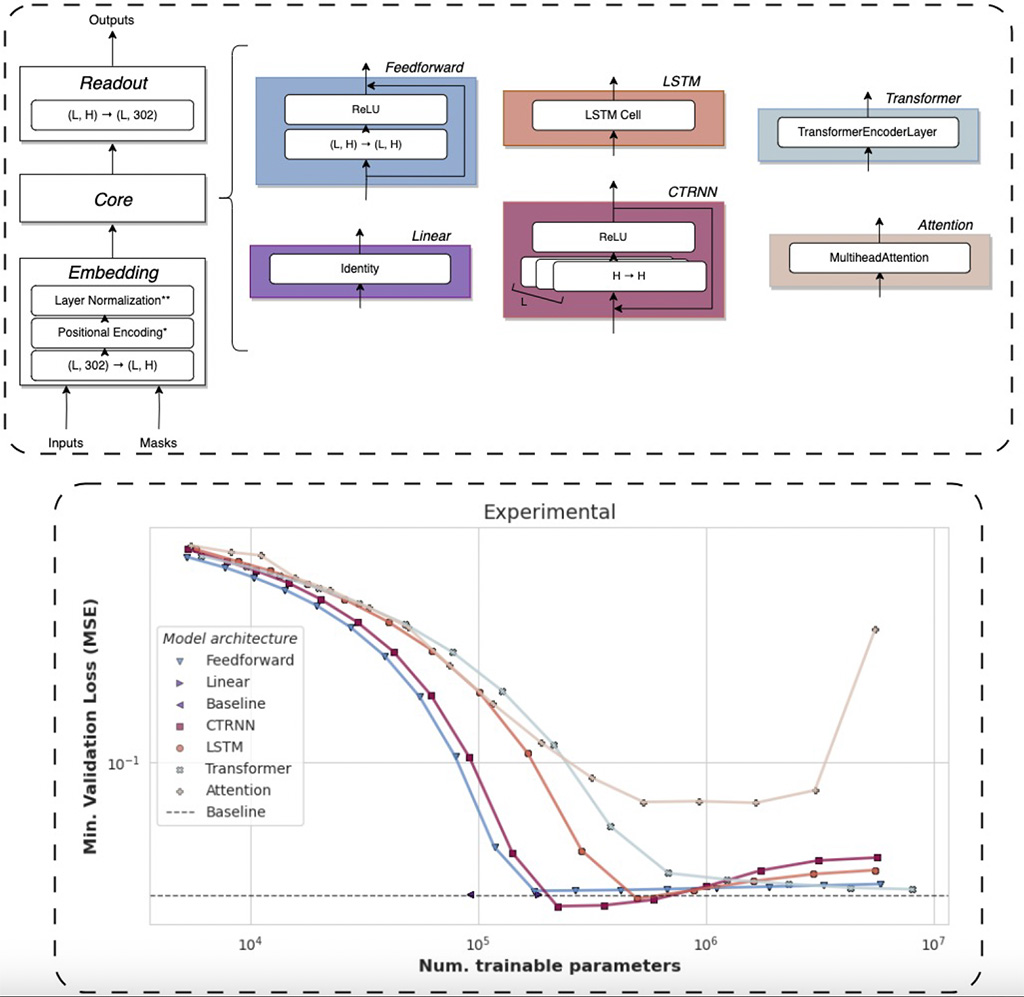
The nematode worm C. elegans provides a unique opportunity for exploring in silico data-driven models of a whole nervous system, given its transparency and well-characterized nervous system facilitating a wealth of measurement data from wet-lab experiments. This study explores the scaling properties that may govern learning the underlying neural dynamics of this small nervous system by using artificial neural network (ANN) models. We investigate the accuracy of self-supervised next time-step neural activity prediction as a function of data and models. For data scaling, we report a monotonic log-linear reduction in mean-squared error (MSE) as a function of the amount of neural activity data. For model scaling, we find MSE to be a nonlinear function of the size of the ANN models. Furthermore, we observe that the dataset and model size scaling properties are influenced by the particular choice of model architecture but not by the precise experimental source of the C. elegans neural data. Our results fall short of producing long-horizon predictive and generative models of C. elegans whole nervous system dynamics but suggest directions to achieve those. In particular our data scaling properties extrapolate that recording more neural activity data is a fruitful near-term approach to obtaining better predictive ANN models of a small nervous system.
Original biorxiv preprint: version 1 posted on February 14, 2024, and available [here]